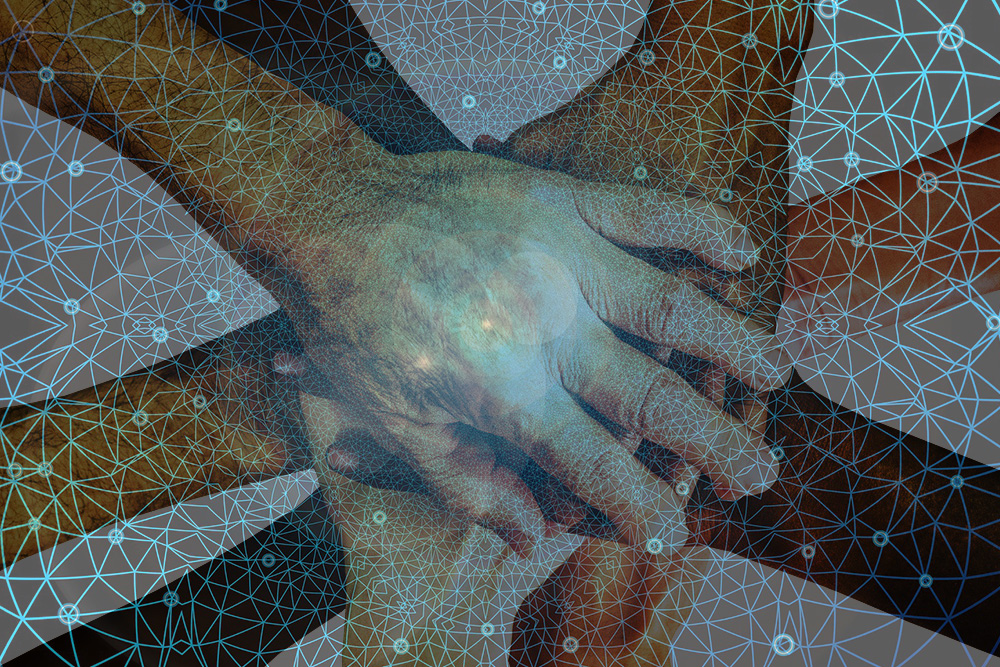
Creating Artificial Intelligence in 'Full Color'
At many American hospitals, bedside monitors that measure everything from intracranial pressure to heart, respiration, and blood pressure rates
“AI informed by data from homogenous populations poorly generalize, which is why diverse approaches in healthcare, AI included, are so critical.”
Ishan Williams, professor, who earned a $5.9M NIH grant to bolster diversity in AI healthcare
feed numbers into AI systems that constantly assesses patients for things like stroke, sepsis, and myocardial infarction.
But because the algorithms that feed predictive healthcare AI systems are often based on homogenous populations (white, urban, college-educated men, for example) as observed by physicians (rather than the entire clinical team), AI that’s meant to improve care may sometimes fall short—and even prove harmful.
With a new $5.9 million National Institutes of Health grant, primary co-investigators Ishan Williams, an associate professor in the School of Nursing, and Randall Moorman, a UVA Health cardiologist, will develop, test, and deploy best practices for artificial intelligence health care systems that aggregate data from a more diverse pool of patients by taking into account their race, ethnicity, socio-economic status, and geography.
[VIDEO] Meet Ishan Williams, PhD, FGSA
In a nation with a growing proportion of racial and ethnic diversity, AI best practices have become a priority for the NIH, which, with its “Bridge to AI” programs, has assembled an array of scholars tasked with its improvement. Williams’s and Moorman’s work will help establish methods to more accurately, fairly, and transparently predict adverse health events, inform treatments, and improve recovery from acute illness based on data from a greater diversity of patient populations.
- read ANIMATING ALZHEIMER'S (VNL winter 2022)
The “Patient-Focused Collaborative Hospital Repository Uniting Standards (CHoRUS) for Equitable AI” grant will develop a publicly available, AI-ready critical care dataset of unprecedented diversity, while outlining best practices for AI that promote privacy, accountability, clinical benefit, and equity to a broad, new generation of AI clinicians and scientists.
“Genetically speaking, human beings are nearly identical,” said Williams, “but there are environmental and geographic factors that can influence the way our genes are expressed, which impact the way we experience chronic conditions and diseases. It’s not until relatively recently that we’ve recognized the impact of racism in health care. We need our AI and the systems that inform it to reflect and account for that understanding.”
“AI importantly informs how we care for critically ill patients, but without diverse data at its core, its relevance is limited. This grant, these scholars, and their work will compel that science forward to ensure not only that AI’s building blocks are diverse, but also that its deployment is equitable, accessible, and ubiquitous.”
Marianne Baernholdt, dean and professor
COVID-19 has proven particularly vexing for communities of color, which have been, compared to their white peers, devastated by the disease. “If COVID has taught us anything,” Williams said, “it’s that we must take a nuanced, inclusive, equitable approach to everything we do in health care, AI included.”
- read WHY COMMUNITY ADVISORY BOARDS? (VNL fall 2022)
Acting as co-primary investigators and module leaders, Moorman and Williams are working with investigators at Massachusetts General Hospital, the University of Florida, and colleagues across the country in biomedical and clinical organizations, industry, and regulatory agencies. In addition to new biomedical and behavioral AI data sets, the NIH grants will create a central repository to integrate activities and knowledge while disseminating knowledge, best practices, and training materials.
“AI importantly informs how we care for critically ill patients,” said Marianne Baernholdt, UVA School of Nursing dean, “but without diverse data at its core, its relevance is limited. This grant, these scholars, and their work will compel that science forward to ensure not only that AI’s building blocks are diverse, but also that its deployment is equitable, accessible, and ubiquitous.”
Williams’s and Moorman’s work began in late 2022; the AI collaborative, training materials, and diverse data sets are expected to be completed by 2027.